A newly released video from the University of Zurich (UZH) that seems right out of science fiction shows an AI-controlled high-speed drone beating the top human racers head-to-head for the first time ever. The potentially landmark achievement has been compared to IBM’s Deep Blue supercomputer beating chess master Gary Kasparov in a game of chess in 1996, once considered the hallmark event in artificial intelligence meeting and exceeding human intelligence.
AI Exceeding Human Capabilities Previously Confined to the Digital Environment
Since Kasparov’s history-making defeat, a number of AI systems have outperformed humans in a wide range of challenges. Most notably, Google’s AlphaGo beat the world’s top Go champion, Lee Sedol, in 2016, a game that is much more complex than chess and one that had proven much harder for AI systems to master.
More recently, AI has proven to be better than humans at creative writing, a skill many believed could be beyond AI’s abilities. Still, all of these accomplishments have taken place in the digital world, whereas efforts to take AI to the real world, namely self-driving cars and fully automated factories, have proven woefully inefficient and far from perfect.
Now, the UZH team says that along with a little help from Intel, their AI system has broken out of the digital environment and beaten the world’s top drone racers on an actual real-world racetrack, potentially marking a significant breakthrough in AI’s ability to meet and exceed human abilities.
High-Speed AI-Controlled Drone Used Simulated Environment to Learn How to Fly
Rather than trying to anticipate every little issue that may challenge their AI drone, dubbed SWIFT, during its race with humans, the UZH researchers instead used a trial-and-error approach called reinforcement learning to teach their AI pilot how to fly. This meant training SWIFT in a simulated environment rather than an actual race course, mostly to avoid constantly damaging the drones in the early phases where crashes are commonplace. Still, the researchers did their best to teach the drone in an environment that simulated much of what it would ultimately encounter in its big race.
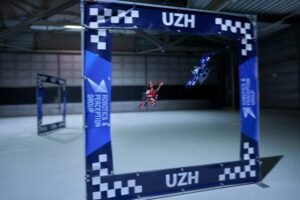
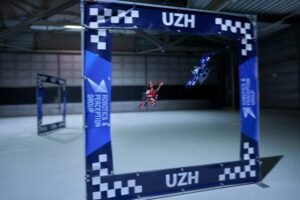
“To make sure that the consequences of actions in the simulator were as close as possible to the ones in the real world, we designed a method to optimize the simulator with real data,” explained Elia Kaufmann, first author of the paper. This included using a precise location signal like GPS to tell the drone where it was, as well as asking the drone to use its onboard camera to detect its environment. Correlating the two data points made it increasingly proficient with the camera and decreasingly dependent on the location signal until it was finally able to race with only the camera input.
Following a month of simulated flight time, which the researchers note “corresponds to less than an hour on a desktop PC,” SWIFT was ready to take on the top three human challengers. In this case, that meant three-time Swiss champion Marvin Schaepper, 2019 Drone Racing League champion Alex Vanover, and 2019 MultiGP Drone Racing champion Thomas Bitmatta. Like SWIFT, the racers also only operated with data from a camera on their drones that was fed to a VR headset, a type of drone racing known as first-person-view (FPV).


Next, the racers all had to navigate a 25-meter x 25-meter track with seven gates that had to be flown through in a particular sequence. Each racer also had to execute some difficult maneuvers, including something called a Split-S that the researchers say “involves half-rolling the drone and executing a descending half-loop at full speed.”
In the video, the incredible speed of the drones, as well as the tight confines under which the maneuvers were executed, are immediately evident, further complicating the task. Nonetheless, SWIFT came out on top, besting the nearest competitor by about one-half of a second.
AI-Controlled Devices Operating in Real World Environments Will Likely Decide Their Utility
In their published paper, the UZH team notes that the AI wasn’t exactly undefeated. For example, when the lighting was changed, or other aspects of the environment were changed so they varied from the one SWIFT had been trained on, the humans came out on top. This is critical, they note, since the ability to effectively interact in the physical world, whether in sport-racing or factory work, will ultimately determine how prevalent AI systems become.
“Physical sports are more challenging for AI because they are less predictable than board or video games,” said Davide Scaramuzza, head of the Robotics and Perception Group at the University of Zurich. “We don’t have a perfect knowledge of the drone and environment models, so the AI needs to learn them by interacting with the physical world.”
The researchers also point out that the ability of their drone to operate at racing speed could add an additional benefit to some real-world uses of AI-controlled drones. This includes potential applications in monitoring forests, law enforcement, traffic management, space flight, or even in action films where AI-operated drone cameras would be able to keep up with the extreme pace of the action being filmed.
“Drones have a limited battery capacity; they need most of their energy just to stay airborne,” said Scaramuzza. “Thus, by flying faster, we increase their utility.”
Christopher Plain is a Science Fiction and Fantasy novelist and Head Science Writer at The Debrief. Follow and connect with him on X, learn about his books at plainfiction.com, or email him directly at christopher@thedebrief.org.